Cause v effect
Because we have finite time and resources to deliver our projects, not every risk can or even should be addressed.
Therefore, in order to arrive at the relative priority of risks, we express our final risk score as a function of cause and effect.
In other words, we rank each risk by multiplying its probability by its impact scores.
For example, let’s say we intend to issue tablet computers to all our project team to work within the field.
We immediately identify the risk that they will be damaged.
Analysis of all possible causes leads us to believe that the likelihood of this is ‘possible’, or 3 out of 5.
The greatest impact (or effect) was estimated to be on the project’s time, which was rated as ‘major’, or 4 out of 5.
Therefore, our risk score = 3 x 4 = 12.
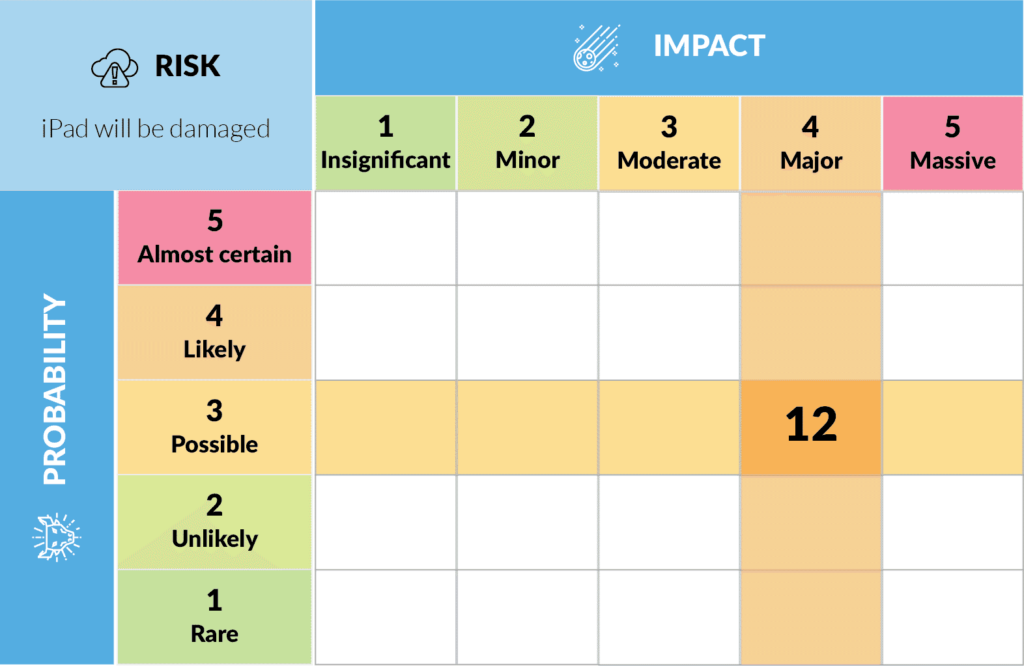
Another example
Here’s another example from the same project…
There is a risk (or opportunity, in this instance), that Ryan Gosling will volunteer to work with us.
Using all the channels available to us, the probability of this occurring is rated as pretty remote (or 1 out of 5); however, the impact on our project and organization’s reputation would be massive (or 5 out of 5).
In this case, our risk score = 1 x 5 = 5.
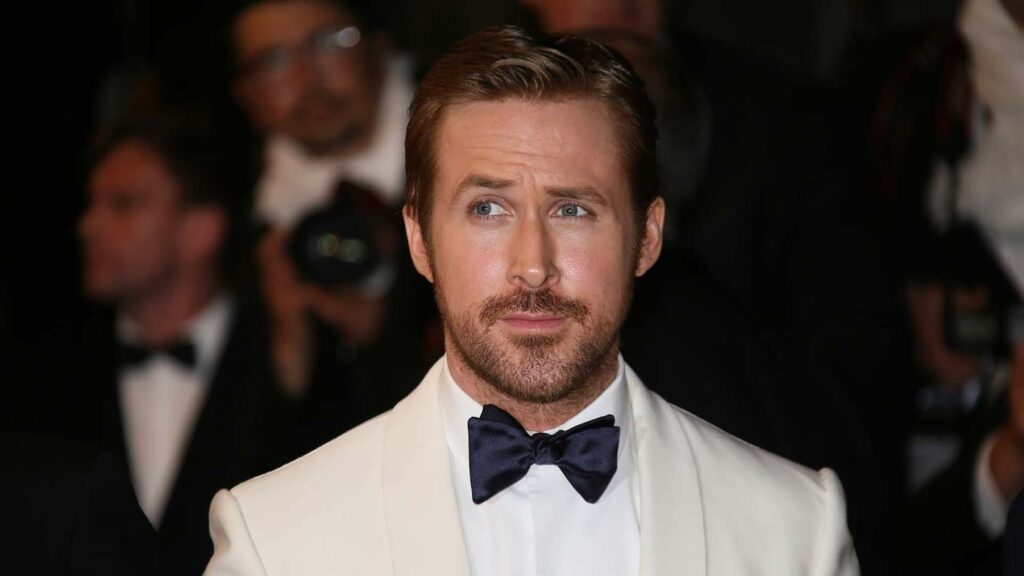
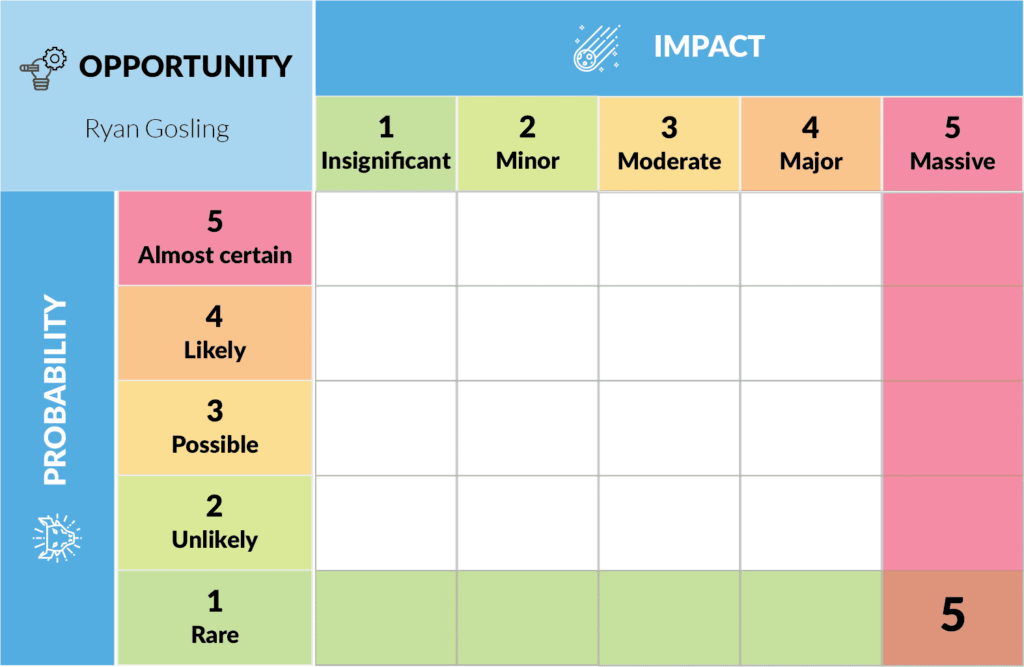
We can immediately see that our iPad risk has a higher rating than our chances of working with Ryan Gosling.
This means that when it comes to treating our risks, which is the next step in the process, we will give higher priority to the iPad event.
Prioritizing risks qualitatively is as simple as multiplying probability by impact, and ranking the outcomes.